Our Automated Valuation Model (AVM) prototype for South Korean Commercial Real Estate (CRE)
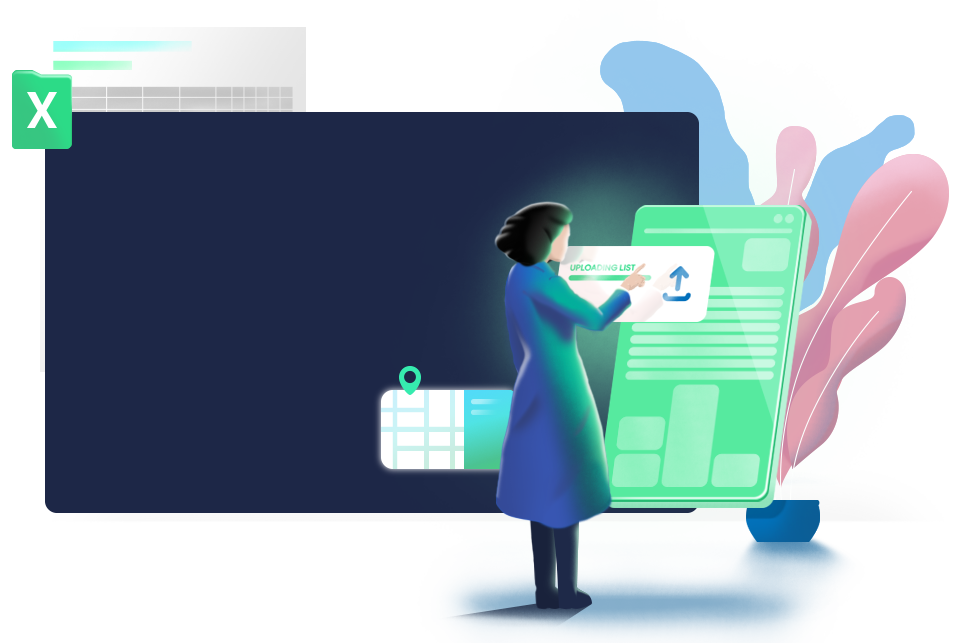
Written by: Raymond Chetti; Co-author: Felipe Ramirez
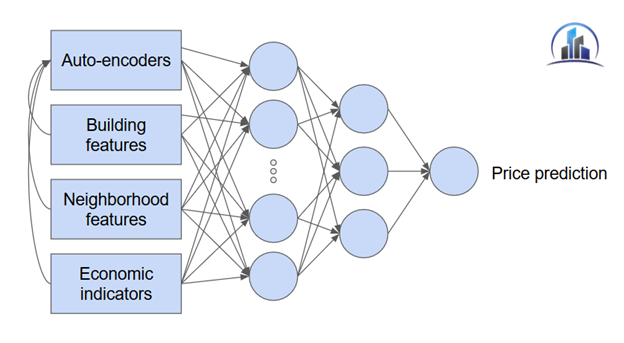
Since getting to know Jane and learning about her vision for GRAS, I hoped our start-up could play a role in her larger vision not only for the South Korean real estate market, but globally, as GRAS has (and is building) relationships in Vietnam, Japan, and the US, in addition to its’ base of 120+ brokers/agents in South Korea.
Within the course of about a month we have been able to develop a functional prototype of one of our core products called the Value Report, which features a front-end and automated valuation model (AVM / 상업용 부동산 자동화 평가모델), which is able to tell us the market value for commercial buildings in our specified region of focus, Gangnam-gu, Seoul.
All any user has to do is enter the building’s address, click enter, and we’re able to generate an automated valuation report within an instant like this:
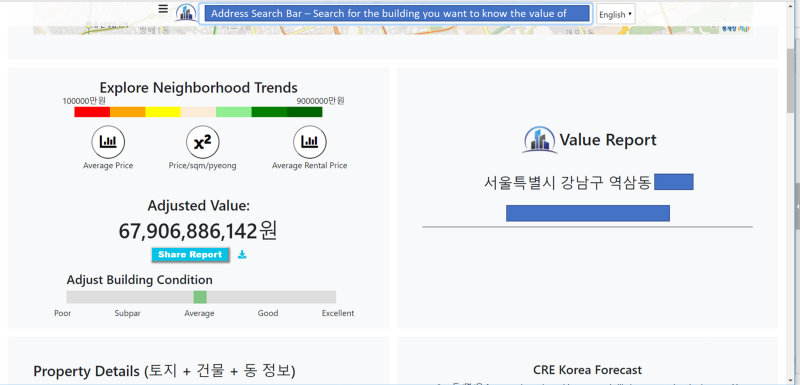
I know I’m not only speaking for myself, but our team at CRE Korea as I say that I’m sincerely looking forward to working with Jane and other GRAS members to refine our work at CRE Korea as we will be enabling GRAS members the opportunity to use our products to close more deals.
We’re excited for what is to come given the short amount of time and resources we’ve been able to dedicate to our prototype’s development.
You might be asking yourself a few questions, a few of which we’re happy to answer below, but for any other questions please feel free to message me on LinkedIn! We’re happy to chat with those who are interested in our work.
1. What is your model’s accuracy?
To date we’ve developed two variations of our AVM, let’s call them v1 (developed August 22, 2018) and v2 (developed August 29, 2018).
Our v1 took about one month to develop (data sourcing, cleaning/pre-processing, and modeling work) and features only about 3–4% of all the big data we’d like to integrate into our AVM.
Within one week of improving the v1 model, we’ve been able to enhance its’ accuracy by lowering our mean absolute error (MAE) by 8% for an updated v2 model.
Our v2 model’s MAE is about 6–13% away from what traditional, human appraiser’s margin of error is for when they appraise properties. Traditional appraisals by humans are typically 7–14% away from actual transaction prices (Kok, et.al, 2017).
Given our rate of progress (data processing, cleaning, and preparation for consumption by our machine learning model) we can anticipate our AVM will appraise and value commercial real estate like a human by the end of September 2018.
2. How do you know your margin of error/accuracy?
We’ve collected actual CRE transaction data for the Korean market from 2006 until July 2018 (updated monthly) and were able to back test our model against these records. Then, we calculated the MAE and the coefficient of determination in order to have a measure of its precision and accuracy.
3. What data are you using and what are your sources?
Since this is part of our “secret sauce”, we’re not inclined to say exactly, but please know this — we are compiling and aggregating one of the largest and most diverse sets of big data in South Korea that will be related to CRE valuation and we’ve just begun.
We’ve identified dozens of data endpoints from over 30+ data sources that are related to CRE given our team’s collective experience in the CRE industry and are working to collect, process, and integrate these endpoints into our machine learning model.
4. What’s next for us?
We’re working to refine our model’s accuracy by pre-processing and preparing more data for the model to consume and learn from.
In addition, we’ll be seeking partners who are interested in helping scale our business (our CRE AVM, products, team) to the entirety of South Korea and potentially other parts of Asia.